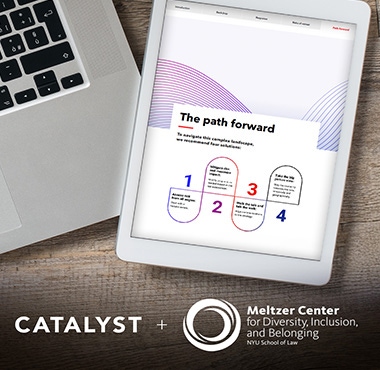
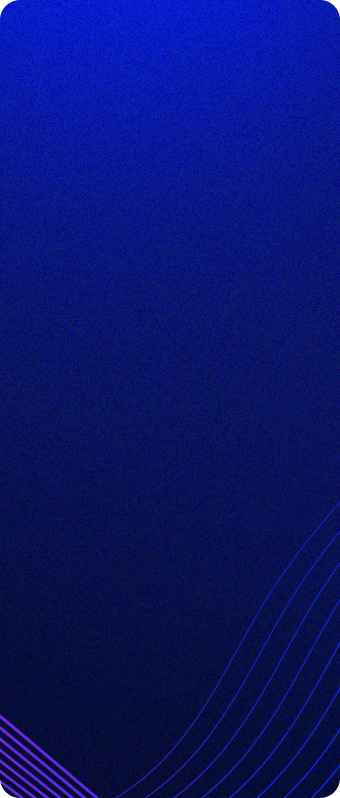
Risks of retreat: The enduring inclusion imperative
Understand the risks of stepping back from inclusive workplace practices.
Understand the risks of stepping back from inclusive workplace practices.
480+
resources to unlock
50k
gender equity advocates
60+
years of proven impact
24M
employees supported
Understand the risks of stepping back from inclusive workplace practices.
Opinion
Guide
Guide
Guide
Gain access to research-backed solutions, events, communities, and insights that drive real business outcomes.
Our research and insights address the most pressing challenges impacting workplaces today.
Tap into a global network of leaders and advocates dedicated to fostering inclusive workplaces.
From expert-led webinars to dynamic conferences, our events inspire action and provide tools to create meaningful change.
Create inclusive workplaces that help you attract and retain talent at all levels with our proven workshops, speakers, and learning programs.
Start shaping the workplace of tomorrow. Supporter companies empower change agents across their organizations with exclusive tools, resources, and programming.
Level up your inclusion and equity initiatives and scale your impact with research-backed tools, solutions, and strategies.
Build programs and strategies that drive real business impact within a shifting legal, political, and economic landscape.
Enhance your inclusive leadership skills and achieve goals like elevating your ERG programming and company culture by learning.
Harness the power of inclusive leadership to inspire followership, foster innovation, increase productivity, and attract top talent.
Prepare your workforce for the future by equipping leaders, managers, and advocates with essential soft skills to build inclusive workplaces.
Catalyst has been a trusted partner in our firm's inclusive and intersectional work to eliminate systemic barriers for women in our workplace and in the communities we serve. Their cutting-edge research supports our work in advancing women into positions of leadership in the legal profession.
Charlene Theodore
Chief Inclusion Officer, McCarthy Tétrault
Catalyst has really leaned in and works with so many of our partner organizations, but also their initiatives really put a heavy emphasis on how we empower women of color to make sure that they are rising up into the C-suite. Catalyst to me is the gold standard of empowerment for underrepresented communities.
Cid Wilson
CEO, HACR
I mean, when you hear something from Catalyst, you know that it's going to be good. You know that there's going to be data and resources to back it up and support it. They are the paramount company when it comes to advancing women in the workplace.
Angela Bretz
SVP, Human Resources Business Partner, Nationwide
We've now put about 400 people leaders -- mostly men, of course, [participate in MARC] as we know the formula: It's about 80% men, 20% women in workshops – and frontline leaders through the workshop. We're seeing a difference, especially in some of the organizations that have put more of their people leaders through.
Vivian Yoanidis
Senior Manager of Diversity, Equity, and Inclusion, Hydro One
One of the ways that Catalyst has really partnered with us is helping us think about what the future of diversity, equity, and inclusion work looks like for us and how we're able to achieve it in a way that gives us a global lens based upon national and truly global best practices.
Davis Bubas
Senior Director, UPMC Center for Engagement and Inclusion
Like many others, we were just not aware of the biases that we had and the impact that has had on women in our workplace…and with that awareness comes understanding, and through understanding, then you’re empowered to act.
Tuong Lee
Technology and Innovation Executive, Enbridge
MARC was an awakening....it equipped me with the skills to feel like I had the courage to engage in these [difficult] conversations and to question everything I knew and that I had been conditioned with.
Alex Lambros
Project Manager, Petroleum Engineer, Global ERG Leader, Chevron
Make diversity and inclusion your strategic advantage. Start creating high-performing workplaces, unlock new levels of productivity, and prepare for what’s next.